Data-Driven Companies Perform Better by Almost Every Metric — And Get More Out of AI

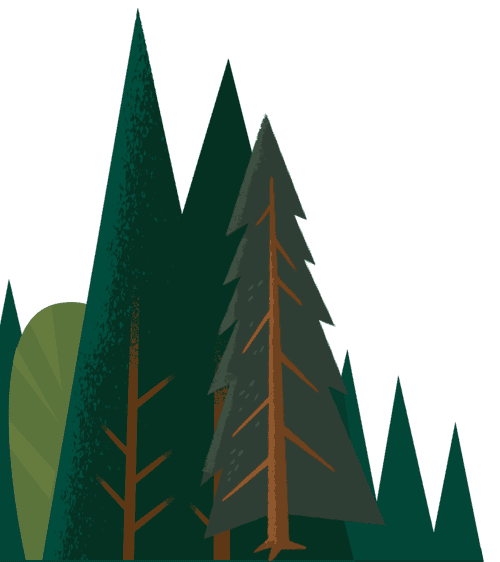

It's time to give everyone in your organization the know-how to use data to make better decisions. Your business depends on it.
Behind the data
Most business leaders say data is critical to decision-making, but a new global survey of 10,000 of them reveals a different story.
- 67% of leaders are not using data for important decisions like pricing
- Fewer than one-third of them use data to inform strategy when entering new markets
- 79% don’t use data to inform their diversity and inclusion policies
- Only 17% use data to help guide their climate targets
The case for change
There are mission-critical insights locked inside your data. AI can help you quickly identify important trends, opportunities, and challenges. But that’s only half the equation. Building a data culture, along with trusted AI, is key to unearthing these buried insights.
- The 58% of companies that make decisions based on data are more likely to beat revenue targets than those that don’t
- Companies that add AI to their data toolkit increase revenue by an average of 30%
- Companies that embrace this approach reallocate talent and capital four times faster, and are able to quickly create unique and personalized customer experiences
What you can do now
Here are some suggestions for building a world-class data culture. We’ll dig deeper into each below.
- Choose the right team members
- Give your team the right training and technology
- Test your assumptions on a small scale and iterate
- Prioritize your data culture’s human element
- Identify where AI can help you get more value from your data
A shared view of customer data helps Formula 1 turn 3x fan growth into lasting loyalty
The next step in Formula 1’s remarkable rise: experiences that feel personal, like a front-row seat on top of your favorite team’s garage. Here’s what that requires.



What’s the difference between being data-driven and data-informed?
In a data-driven organization, all or most employees can find and analyze data, extrapolate what it means, build a dashboard, and use data to decide next steps. Employees don’t rely on data analysts to do this.
Being data-informed means making decisions based on a mix of data, internal research, personal experience, and insights. Data-informed organizations may or may not possess the data skills of data-driven ones.
Why building a data culture is essential
CEOs face countless decisions about where to start when building a data culture. Overcome analysis paralysis by starting small with a use case that proves the value of your new data culture. Promote the payoff with skeptics: McKinsey research shows data-driven companies accomplish goals faster and that their initiatives contribute at least 20% to earnings before income taxes.
Here’s why this works:
Data analysis identifies actionable trends
Data analysis surfaces patterns that unearth value, and enables companies to take advantage of market opportunities faster. Adding AI to the mix can speed up the process even more by analyzing the data at scale and providing recommendations. Combining the two drives growth, nurtures innovation, and strengthens differentiation from competitors.
Artificial intelligence and machine learning supercharge success by 30%
Companies that still rely on institutional knowledge and gut feelings to guide decision- making are leaving money on the table by missing opportunities. With AI and machine learning, employees make the right decisions quickly and confidently. Adding AI to CRM systems across business functions takes the guesswork out of decision-making and boosts success by an average of 30% (according to Salesforce research) across key metrics like efficiency, productivity, and revenue growth.
Strategic work keeps employees engaged
When data analyses guide routine decisions, employees spend less time on basic tasks that add no value, and more time focusing on strategic work. That keeps them engaged and productive. That’s why 84% of data-leading organizations have observed an increase in employee retention.
Empower the right team to score a financial win
The best way to build a community of data champions is to demonstrate how data-driven decision-making grows revenue and streamlines operations. Don’t choose an analytics use case just because it might produce an interesting outcome. Instead, opt for a project that will yield a financial win and that you can scale for maximum impact.
Here’s how to start:
Step 1: Choose the right team members
Create a working group of diverse colleagues from across the organization. These team members should bring a collaborative mindset, differentiated skills and abilities, and distinct organizational perspectives. Make sure you include executives, line managers, data engineers, developers, and machine learning architects.
Step 2: Equip your team with the right training and technology
The stats on data literacy are not great. Only 35% of workers say they have been trained on data visualization tools, and 29% on statistical tools. Twenty-seven percent say they are able to read data outputs relevant to their role, and only 26% say they can make decisions based on data. With technology powered by augmented analytics, you can enable all workers to unlock the power of data from insight to action.
Step 3: Start small
Test your assumptions on a small scale and iterate. You’ll know you’ve hit a winner when your colleagues can measure the value of your project on their bottom line.
Here’s how this worked at one financial services company. After a simple clustering analysis evaluated smaller classes of data across sales territories, right-sizing the coverage led to $1 million in incremental revenue the following year. That win was enough to build enthusiasm for data-driven decision-making across the company.
Step 4: Prioritize the human element of a data culture
Ensure team members are involved in the set up, testing, tuning, and application of analytics to avoid bias and errors — which can have a negative effect beyond just profits.
Avoid bias by proxy by not taking data at face value. For example, ZIP codes: at face value, they are nothing more than a location indicator. But when you consider ZIP codes are often a proxy for an area’s racial makeup — and lenders and insurers consider ZIP codes in loan applications — human reviewers must step in to ensure decisions made based on this data point are fair and free of bias.
Step 5: Identify where AI will help your business
There are many places to start on your AI journey, or continue it if you’ve already begun. Establish ethical standards, consistency, and accountability from the start. Getting your data ready at the outset will ensure you make the best use of AI.
Take the next step to build your data culture
Look, this is hard stuff. These things don’t happen overnight. This type of transformation may be new for many business leaders. As you embark on your new approach, keep in mind that it will take time, trial and error, and a culture shift. However, leading companies encourage experimentation because they believe that not being data-driven and embracing AI is the bigger institutional hazard. And inaction is the biggest risk of all.