AI Is Everywhere — But Are You Building it Responsibly?

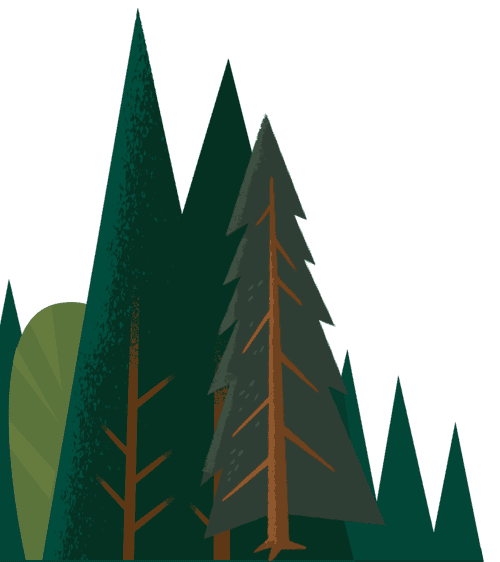

The biggest barrier to widespread artificial intelligence isn’t immature technology — it’s skepticism and lack of trust. Here’s what business leaders can do about it.
Artificial intelligence (AI) touches billions of people daily. It suggests recommended content on your favorite streaming service and helps you avoid traffic while your drive. AI can also help businesses predict how likely someone is to repay a loan or, in today’s period of supply chain disruption, determine the most efficient routes for distributors to ship goods quickly and reliably.
There is no doubt the predictive capabilities brought about by these algorithms have helped businesses to scale rapidly. With applications in fields from medical research and retail services to logistics and personal finance, the global AI industry is projected to reach annual revenue of $900 billion by 2026, according to IDC. But for all the good AI has contributed to the world, the technology isn’t perfect. The AI algorithm can cause many business and societal fouls if not kept in check.
AI is trained on reams of data that have been collected over time. If that data is collected shows bias or is not representative of the people the system will impact, it can amplify those biases. For example, popular image dataset ImageNet initially perpetuated negative biases by mislabeling or identifying people incorrectly in internet image searches. Research has shown that many popular open-source benchmark training datasets — ones that many new machine learning models are measured against — are either not valid for the context in which they have been widely reused or contain data that is inaccurate or mislabeled.
Even the way AI is applied can magnify existing inequities in society. For example, more surveillance technology tends to exist in neighborhoods with large populations of people of color. This can result in large numbers of arrests of people of color in those neighborhoods, even if the same crimes occur at the same rates in predominantly white neighborhoods.
Innovate with AI
Einstein AI helps to empower your teams with built-in intelligence to engage with empathy, increase productivity, and scale customer experiences.



Ways to combat bias in AI
As a result, governments around the world have begun drafting and implementing a bevy of AI regulations. Since 2019, at least 17 U.S. states have proposed bills or resolutions to regulate AI applications. The debate about regulating AI, though, is global, and heated up in 2021. India, China, and the European Union all published guidance or draft regulation for responsible AI last year. All of these discussions are happening as governments increasingly employ AI in public governance and decision-making.
Regulation, when well-crafted and appropriately applied, will force the worst actors to do the right thing. In the meantime, business leaders need to pave the way to a more equitable AI infrastructure. Here’s how:
Be transparent
To increase trustworthiness, many regulations require businesses to be transparent about how they trained their AI model (a program trained on a set of data to recognize certain types of patterns), the factors used in the model, its intended and unintended uses, and any known bias. This is usually requested by policymakers in the form of data sheets or model cards, which act like nutrition labels for AI models. Salesforce, for example, publishes its model cards so customers and prospects can learn how the models were trained to make predictions.
Make your AI interpretable
Why does an AI system make the recommendation or prediction it does? You might shrug if AI recommends you watch the new “Top Gun,” but you’ll definitely want to know how AI weighed the pros and cons of your loan application. Those explanations need to be understood by the person receiving the information — such as a lender or loan officer — who then must decide how to act upon the recommendation an AI system is making.
That said, one study conducted by researchers at IBM Research AI, Cornell University, and Georgia Institute of Technology found that even people well versed in AI systems often over-relied on and misinterpreted the system’s results. Misunderstanding how the AI systems work “can lead to disastrous results and over- or under-confidence in situations where more human attention is warranted,” the research noted. The bottom line? More real-life testing needs to occur with the people using the AI to ensure they understand the explanations.
Keep a human in the loop
Some regulations call for a human to make the final decision about anything with legal or similarly significant effects, such as hiring, loans, school acceptance, and criminal justice recommendations. By requiring human review rather than automating the decision, regulators expect bias and harm to be more easily caught and mitigated.
Unfortunately, humans aren’t generally up to the task. Human nature drives people to take the path of least resistance, so they usually defer to AI recommendations rather than rely on their own judgment. Combine this tendency with the difficulty of grasping the rationale behind an AI decision, and humans don’t actually provide that safety mechanism against bias.
That doesn’t mean high-risk, critical decisions should simply be automated. It means we need to ensure that people can decipher AI explanations and are incentivized to flag a decision for bias or harm. The General Data Protection Regulation (GDPR), the EU’s law on data protection and privacy, allows AI to make recommendations for decisions with legal impact (for example, a loan approval or rejection) but requires a human to make the final decision.
Avoid proxy variables
Legally, hiring or loan decisions cannot be based on someone’s age, gender, race, or other protected attributes, regardless if a human or AI is making the decision. As a result, those attributes shouldn’t be included in the model. But it also means the model should avoid proxy variables, which are highly correlated with the sensitive variables. For example, in the U.S., postal zip code is highly correlated with race, so if the model excludes race but includes zip code, it likely still includes bias.
How to add ethical AI practices to your business
It’s understandable, then, that some executives may be reluctant to collect sensitive data — age, race, and gender, for example — to begin with. Some worry about inadvertently biasing their models or being unable to properly comply with privacy regulations. However, you cannot achieve fairness through inaction. Companies need to collect this data in order to analyze if there is disparate impact for different subpopulations. This sensitive data can be stored in a separate system where ethicists or auditors can access it for the purpose of bias and fairness analysis.
In a perfect world, AI systems would be built without bias, but this is not a perfect world. While some guardrails can minimize the effects of AI bias on society, no dataset or model can ever be truly bias free. Statistically speaking, bias means “error,” so a bias-free model must make perfect predictions or recommendations every time — and that just isn’t possible.
There is a lot to understand to ensure companies are creating and implementing AI responsibly and in compliance with regulations. This requires business leaders to build ethical AI practices.
Executives can start by hiring a diverse group of experts from many backgrounds in ethics, psychology, ethnography, critical race theory, and computer science. They can build on that by creating a company culture that rewards employees for flagging risks — empowering them to not only ask, “Can we do this?” but also, “Should we do this?” — and by implementing consequences when harms are ignored.
Get a 360° view of success
Vantage Point magazine brings together thoughtfully curated voices from the world’s most influential companies. Each issue unpacks intimate insights shaping the workplace.


