Is Your Marketing Working? Good Data Correlation Will Tell You

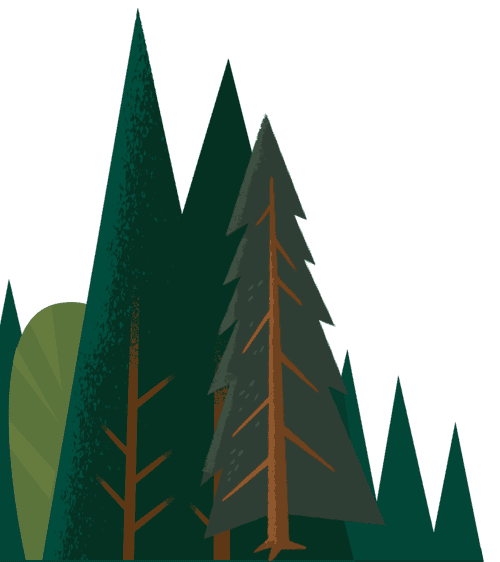

These tips can help you avoid common mistakes when trying to show correlation or causality in marketing data sets.
It’s the million dollar question: How do I know if my marketing is persuading my audience to take action? The answer lies in good data correlation.
Data correlation is a statistical measure that describes the relationship between two or more things. For example, how does a person’s weight correlate with what they eat? How does a marathon runner’s race time correlate with the number of hours they train?
Causation is the term for showing the impact of one thing on another thing. A runner trains 25 hours a week, so his race time dropped by a minute.
In marketing, we seek to find and act on correlations and causations.
Turn marketing data into action
Learn the three steps to success: analysis, optimization, and alignment.



How does data correlation work in marketing?
Any data can be correlated to show a relationship. However, that does not necessarily mean one has had an impact on the other.
Marketers need to have an accurate view of the impact of their messages, campaigns, and media spend. The more insight they gain, the greater impact marketers have both on their customers and their business.
We talk about correlation and causation in marketing all the time. Did our latest campaign boost sales? Did our Twitter ad increase awareness? Can we prove that one thing caused another?
Here are some key tips to avoid easily made mistakes when trying to show correlation or causality in marketing data sets:
1. Avoid inflammatory statements
One of the greatest traps in data correlation is the use of inflammatory or sensationalist descriptions to prove a tenuous relationship. Avoid this at all costs. Your executives will see straight through it.
Here’s an example of an inflammatory statement that may mislead data analysis:
“The sunny weather caused a drop in in-store sales”.
While the weather may have an impact on sales performance, it likely was not the only factor. So, the statement is not definitively true and may discredit your data analysis.
Instead, ask yourself these questions to make room for investigation when analyzing data:
- What if we cut the data differently?
- What if we removed particular channels?
- Are there too many variable changes to accurately see a correlation or causation?
- What assumptions are we making?
- Was this intentionally designed (i.e. planned campaign) or a byproduct of other activity?
2. Choose the right graph for your analysis
Within the Marketing Cloud Intelligence platform, you can choose from more than 100 widgets to effectively visualize your data. You will receive suggestions for the appropriate widget to use, according to the data set you’re analyzing. You can also create your own custom widgets to visualize data in your own unique way.
Some suggested graphs to start your correlation analysis include:
Time Series Graphs. These compare multiple metrics over a period of time. They are great for looking at trends and seasonality in data.
Distribution Graphs. Graphs that can easily demonstrate whether there is a correlation. These are great for seeing distribution against a mean.
Relationship Graphs. Graphs that show a relationship between two or more variables. A bubble chart is best used for showing a relationship between three variables.
When sharing your findings, only show the most simple graphs to demonstrate your points. Your homework is important, but not for the executive summary.
3. Use a robust data set for more informed analysis
When analyzing a campaign’s impact on brand KPIs or sales, it’s tempting to use an outlier as the backbone for your impact analysis. For example, one common outlier is an increase in budget, which manipulates numbers. Consistent correlation provides a far stronger argument for analysis.
When interrogating outliers, analyze the integrity of that outlier through rigorous questioning. Is there a cause-and-effect relationship here, or did the numbers simply go up due to an increase in budget? Call out your assumptions.
4. Source reputable data sources
Partial data sets and non-reputable data sources are dangerous.
Partial data sets may provide further context into your findings but shouldn’t form the basis of analysis because they can lead to data bias. Selection bias happens when analyzing a data set that’s not representative of the population. For example, data on sales in one market is not necessarily representative of performance in all markets.
5. Limit variables for testing
Many marketers are in the test-and-learn phase and are constantly optimizing across many tactics and channels. As a result, it can be difficult to isolate the impact of each campaign activity on hypotheses or KPIs.
To further understand the correlation effect, a designed test-and-learn campaign is a great place to start, where you limit variable testing and analyze these isolated results.
6. Always start with a hypothesis
Think of correlation analysis as an experiment. Knowing what your hypothesis is from the start will help you choose the right visuals in aiding your experiment. Often, marketers dive into data without knowing what they want to get out of it, which can lead to an incoherent story.
7. Short-term and long-term measurement
Brands aren’t built overnight. Advertising may or may not have an immediate impact on your brand KPIs. Understanding how your consumers digest the media you put in front of them will help you devise better ways of analyzing your data. A short-term and long-term measurement strategy is useful here.
The key to analyzing correlation and causality is to understand the difference between the two. Start your analysis with correlation, and let your questions guide your findings. May your assumptions always be called out, and your graphs be simple yet effective.
Go forth and correlate.