FAccT 2022 CRAFT Summary: Challenges in FAccT from Research to Practice to Policy

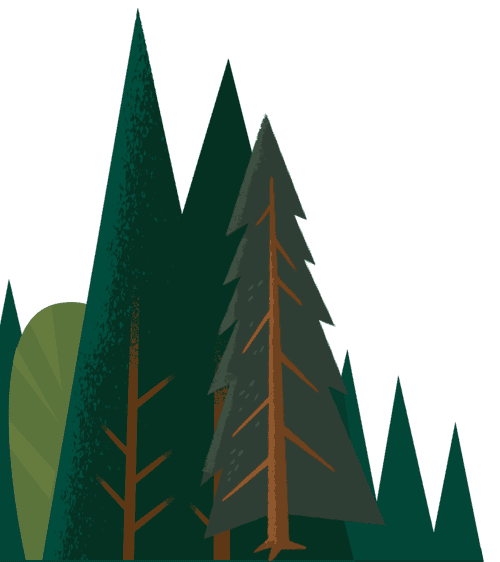

We summarize insights from our FAccT 2022 CRAFT workshop that focused on Concepts of Fairness & Transparency, Applied RAI Practices, Organizational Approaches to RAI & Cultural Change, and Public Policy & Regulation.
Kathy Baxter, Principal Architect, Salesforce & Chloe Autio, Advisor & Sr. Manager, The Cantellus Group
Originally published on August 31, 2002 at https://facctconference.org/2022/outputs.html

Abstract
Twenty-nine AI ethics practitioners, researchers, and students shared their research, experience, and ideas regarding the most challenging emerging problems in the field of responsible AI (RAI) today across four topics: Concepts of Fairness and Transparency, Applied RAI Practices, Organizational Approaches to RAI and Cultural Change, and Public Policy and Regulation. Four key themes emerged from the session:
- Paradoxes abound and require us to balance the tensions between them (e.g., explainability vs. interpretability, transparency vs. privacy, fairness vs. awareness). There are many solutions available today (e.g., privacy-enhancing technologies, robustness testing, adversarial and counterfactual testing) but the more we learn, the more questions emerge about the benefits, risks, and shortcomings of each.
- Significant challenges remain in how to evaluate, monitor, and repair models. We need new, less biased, more representative benchmarks for quality modeling. Synthetic data can help, but questions remain about its capability and applicability. Failure explainability and solid post-deployment monitoring are essential for promoting fair, accurate, and robust AI.
- Creating a mature RAI culture requires similar commitments and resources as those for security, privacy, or accessibility. These resources include comprehensive employee education and empowerment, access to tools and resources for assessments and documentation, champions throughout the organization, a robust incentives structure, and a consistent, scalable auditing process. One tool we need to learn more about is the ethical advisory council since little has been shared by organizations that use them.
- There is a need for public/private collaboration to create standards and regulations. Serious investment in diversity, equity, and inclusion rather than just lip service are also required for meaningful and rigorous RAI practices. And we must take a socio-technical approach to the creation of standards, practices, and regulations.
Background
The 3.5-hour CRAFT session was organized and facilitated by Kathy Baxter, Principal Architect of Ethical AI at Salesforce and Chloe Autio, Advisor and Senior Manager at The Cantellus Group. Sixteen experts shared their insights on emerging challenges from across our field. Twenty-four attendees and three documentarians representing 16 private companies, 17 universities, two government agencies, and two non-profits participated in the discussions.
Themes
Paradoxes abound but so do solutions
Tension exists between many responsible AI concepts (e.g., explainability vs. interpretability, transparency vs. privacy, fairness vs. awareness). For example, explainability and interpretability underpin trust but not every actor is well-intentioned; greater explainability can sometimes undermine safety, as bad actors can learn how to exploit systems.
Additionally, explainability is difficult to define and operationalize since the need for and level of explainability varies in different contexts. For example, “What did it do?” is very different from “Is what it did reasonable?”. And, “Why did X happen?” is very different from “What can I change about X to get a different result in the future?” Audiences may differ in their approaches to those questions in different contexts and how they evaluate values-tradeoffs.
While paradoxes abound, so do solutions (e.g., privacy-enhancing technologies, participatory design, audits, robustness testing, adversarial and counterfactual testing, bias assessments, model cards, fact sheets). These options may be overwhelming, though, as each solution requires a greater commitment beyond what is most obvious or expedient, and many of these solutions need professional or operational help to fully integrate – they are not “drag and drop.” One must begin by making small improvements, even if not a complete solution. Measure or test what is possible initially, because you can’t test for everything. This is where tensions again appear and some tradeoffs must be made (e.g., between fairness, explainability, and robustness).
Significant challenges remain in how to evaluate, monitor, and repair models
Today and historically, bias in datasets is a core concern for the AI ethics community at large. But evaluating, monitoring, and repairing those datasets is an ever-present challenge. Most AI development teams still lack visibility into their models including:
- Explanations of model behavior
- Understanding feature impact and fairness
- Monitoring for potential bias or drift (model decay)
Automated testing has additional challenges including limited testing data, limited variation in the test data, and the fact that independent testers/auditors need fresh, unused test data. Many benchmarks used in models today are not inclusive of everyone impacted (e.g., measuring skin tone in computer vision, Natural Language Processing (NLP) datasets rife with gender or racial bias). Synthetic data can help address some of these issues, but there is debate about its privacy-preserving ability and the utility of the data that may not retain critical signals for accuracy.
Monitoring deployed ML models is as important as validating the models pre-deployment, not only from the perspective of model quality degradation but also from dimensions such as bias/fairness, accuracy, robustness, privacy, safety, and explainability. Model explainability is frequently discussed, but failure explainability is equally important because it is needed to debug and fix a model.
We know what it takes to create a mature RAI culture but we don’t know enough about Ethical Advisory Councils
As indicated in the Ethical AI Maturity Model and noted by some speakers, one of the first steps in creating a responsible AI practice is to create a code of ethics or guiding principles that employees know, understand, and uphold. Employee training was widely implemented by most of the organizations represented in the session but one key question is how to measure the effectiveness of a training program. RAI Champions were also mentioned as a component of a successful RAI practice.
Employees can go through ethics training but if the culture, framework, and tools aren’t there to support the work, it won’t matter and can result in “organ rejection” (as described by one speaker). This dynamic can result in ethics washing and people going through the motions to get past “checkboxes” as quickly as possible This raised the question of what incentives actually work to align people around the need for RAI. Each organization is different, but some common incentives work across many companies and audiences.
Some companies have Ethics Advisory Councils, with make-ups that vary. Little has been published about Ethics Councils, including success metrics, but the primary purpose is usually to invite a diverse range of expertise to guide ethical use decisions. Ethics isn’t something one person or company can decide alone; we need all perspectives and people to ask tough questions and provide counsel. Sharing of best practices and lessons learned regarding the use of Ethics Councils would benefit the field.
Standards-setting and regulation creation require public/private collaboration
Developing AI governance standards and regulation is difficult. Uncertainty and misalignment of definitions for basic yet complicated terms (e.g., AI, fairness, explainability) is one problem. However, participants recognize that context is key here. There is no one-size-fits-all approach to regulating AI because AI is not a monolithic technology, and it will continue to evolve and grow.
Fortunately, many initial regulatory and standards initiatives have been largely collaborative between private and public sectors, and internationally (e.g., the National AI Advisory Council in the US, Singapore’s Ethical Use of AI and Data Advisory Council). Not only will collaboration result in more comprehensive standards and regulations, it will also increase trust in proposed regulations if they are multistakeholder in nature.
The importance of a multidisciplinary approach to regulation cannot be overstated. We are engaging with and trying to manage socio-technical systems, and that requires cross-collaboration. Much research has been published on the impact diverse employee bases and executive teams have on business outcomes. Similarly, a lack of diversity and inclusion has been shown to be a root cause of many harms. There is an epistemic advantage to DEI in AI development that fosters competitive advantage.
Of course, simply having a diverse team is not enough. These teams must be supported by consistent processes that make it easy to do the right thing and difficult (or impossible) to do the wrong thing. Additionally, there is a need for accountability when these processes are not followed and people must be willing to do the work especially when it is not easy. Gratefully, this session demonstrates that we are part of a community that is committed to tackling new problems in this space as they arise, and in a collaborative way.
Conclusion
Many of the RAI practitioners that have been participating in workshops like this one since 2018 noted the increasing number of organizations with RAI teams, the sophistication of questions being asked, and the number of success stories being shared. Although the number of headlines about harmful AI has increased rather than decreased over the years, attendees felt more confident that we are closer to accepted standards for RAI and that we have an even larger community of practitioners to learn from. We encourage everyone to share their work and lessons learned, as well as to participate in public-private collaborations like the development of NIST’s AI Risk Management Framework. You can sign up to receive email notifications about NIST’s AI activities here.