The Rise of Large Action Models Heralds the Next Wave of Autonomous AI

AI agents and assistants have the ability to take action on a user’s behalf, but each serves a distinct purpose.
Generative AI has officially entered its second act, driven by a new generation of AI agents capable of taking action just as deftly as they can hold a conversation. These autonomous AI systems can execute tasks, either in support or on behalf of humans through their ability to leverage external tools and access up-to-date information beyond their training data.
Like an LLM’s more sophisticated cousin, these agents are powered by Large Action Models — the latest in a string of innovations that inch us closer to an autonomous AI future. July saw the release of our small agentic models, xLAM-1B (“Tiny Giant”) alongside xLAM-7B.
xLAM is capable of carrying out complex tasks on behalf of its users, with benchmark testing showing that it verifiably outperforms much larger (and more expensive) models despite its remarkably small size. LAMs offer an early glimpse of a near future where AI-powered agents will extend what we’re capable of as individuals and supercharge the efficiency of organisations. How will this work in practice?
At Salesforce, we believe autonomous enterprise AI will take two primary forms: AI assistants and AI agents. Both share two important traits. The first is agency, or the ability to act in meaningful ways, sometimes entirely on their own, in pursuit of an assigned goal. The second is the remarkable capability to learn and evolve over time, but in distinct ways. AI assistants will adapt in unique, individually tailored ways to better understand a single user – the human user they need to provide assistance for.
AI agents, on the other hand, will adapt to better support a team or organisation, learning best practices, shared processes and much more. Simply put, AI assistants are built to be personalised, while AI agents are built to be shared (and scaled). Both promise extraordinary opportunities for enterprises.
The power of learning over time
The notion of learning and improving through repetition is a fundamental aspect of autonomous AI, but crucial differences exist between different implementations. In the case of the AI assistant, learning is all about developing an efficient working relationship with the human it’s supporting.
Over time, the assistant will identify habits, expectations, and even working rhythms unique to an individual. Given the sensitive nature of this type of data, privacy and security are non-negotiables — after all, no one wants an assistant they can’t trust, no matter how good it is.
AI agents, on the other hand, are meant to learn shared practices like tools and team workflows. Far from being private, they’ll disseminate the information they learn to other AI agents throughout their organisation. This means that as each individual AI agent improves its performance through learning and field experience, every other agent of that type should make the same gains, immediately.
Both AI agents and assistants will also be able to learn from external sources through techniques such as retrieval augmented generation (RAG), and will automatically integrate new apps, features, or policy changes pushed across the enterprise.
Driving real-world impact
Together, agents and assistants add up to nothing less than a revolution in the way we work, with use cases ranging from sales enablement to customer service, to full-on IT support. Imagine, for example, a packed schedule of sales meetings, ranging from video calls to in-person trips across the globe, stretching across the busiest month of the season.
It’s a hectic reality for sales professionals in just about every industry, but it’s made far more complex by the need to manually curate the growing treasure trove of CRM data generated along the way. But what if an AI assistant, tirelessly tagging along from one meeting to the next, automatically tracked relevant details and precisely organised them, with the ability to answer on-demand questions about all of it? How much easier would that schedule be? How much more alert and present would the salesperson be, knowing their sole responsibility was to focus on the conversation and the formation of a meaningful relationship?
What’s especially interesting is visualising how this all would work. Your AI assistant would be present during each meeting, following the conversation from one moment to the next, and developing an ever-deeper understanding of your needs, behaviour, and work habits — with an emphasis, of course, on privacy.
As your AI assistant recognises the need to accomplish specific tasks, from retrieving organisational information to looking up information on the internet or summarising meeting notes, it would delegate to an AI agent for higher level subtasks, or invoke an Action for single specific subtasks, like querying a knowledge article. It might look something like this:

It’s not hard to imagine how AI agents and assistants could benefit other departments as well, such as customer service. For even a small or medium-sized business, the number of support tickets a typical IT desk faces throughout the day can be staggering.
While human attention will always be required for solving complex and unusual challenges that demand the fullness of our ingenuity, the vast majority of day-to-day obstacles are far less complicated. AI agents can take on much of this work, seamlessly scaling up and down with demand to handle large volumes of inbound requests, freeing up overworked IT professionals to focus on tougher problems and reducing wait times for customers.
The challenges ahead
The road to this autonomous AI future won’t be easy, with technical, societal and even ethical challenges ahead. Chief among them is the question of persistence and memory. If we wish, AI assistants will know us well, from our long-term plans to our daily habits and quirks. Each new interaction should build on a foundation of previous experiences, just as we do with our friends and coworkers.
But achieving this with current AI models isn’t trivial. Compute and storage costs, latency considerations, and even algorithmic limitations are all complicating factors in our efforts to build autonomous AI systems with rich, robust memory and attention to detail.
We also have much to learn from ourselves; consider the way we naturally “prune” unnecessary details from what we see and hear, retaining only those details we imagine will be most relevant in the future rather than attempting unreasonable feats of brute force memorisation. Whether it’s a meeting, a classroom lecture, or even a conversation with a friend, humans are remarkably good at compressing minutes, or even hours of information into a few key takeaways. AI assistants will need to have similar capabilities.
Even more important than the depth of an AI’s memory is our ability to trust what comes out of it. For all its remarkable power, generative AI is still often hampered by questions of reliability and problems like “hallucinations”. Because hallucinations tend to stem from knowledge gaps, autonomous AI’s propensity for continued learning will play a role in helping address this issue, but more must be done along the way.
One measure is the burgeoning practice of assigning confidence scores to LLM outputs. Additionally, retrieval augmented generation (RAG) is one of a growing number of grounding techniques that allow AI users to augment their LLM prompts with relevant knowledge to ensure the model has the necessary context it needs to process a request.
Ethical considerations will be similarly complex. For instance, will the emergence of autonomous AI systems bring with them the need for entirely new protocols and norms? How should AI agents and teams talk to each other? How should they build consensus, resolve disputes and ambiguities, and develop confidence in a given course of action? How can we calibrate their tolerance for risk or their approach to conflicting goals like expenditures of time vs. money?
And regardless of what they value, how can we ensure that their decisions are transparent and easily scrutinised in the event of an outcome we don’t like? In short, what does accountability look like in a world of such sophisticated automation?
One thing is for sure — humans should always be the ones to determine how, when and why digital agents are deployed. Autonomous AI can be a powerful addition to just about any team, but only if the human members of that team are fully aware of its presence and the managers they already know and trust are fully in control.
Additionally, interactions with all forms of AI should be clearly labeled as such, with no attempt — well intentioned or otherwise — to blur the lines between human and machine. As important as it will be to formalise thoughtful protocols for communication between such agents, protocols for communication between AI and humans will be at least as important, if not more so.
Conclusion
As ambitious as our vision of an agent-powered future may seem, the release of xLAM-1B Tiny Giant and others in our suite of small agentic models, are strong evidence that we’re well on our way to achieving it.
Much remains to be done, both in terms of technological implementation and the practices and guidance required to ensure AI’s impact is beneficial and equitable for all. But with so many clear benefits already emerging, it’s worthwhile to stop and smell the roses and appreciate just how profound this current chapter of AI history is proving itself to be.
Are you ready for AI?
Take our free assessment today to see if your company is ready to take the next step with AI. You’ll receive customised recommendations, helping you effectively implement AI into your organisation.


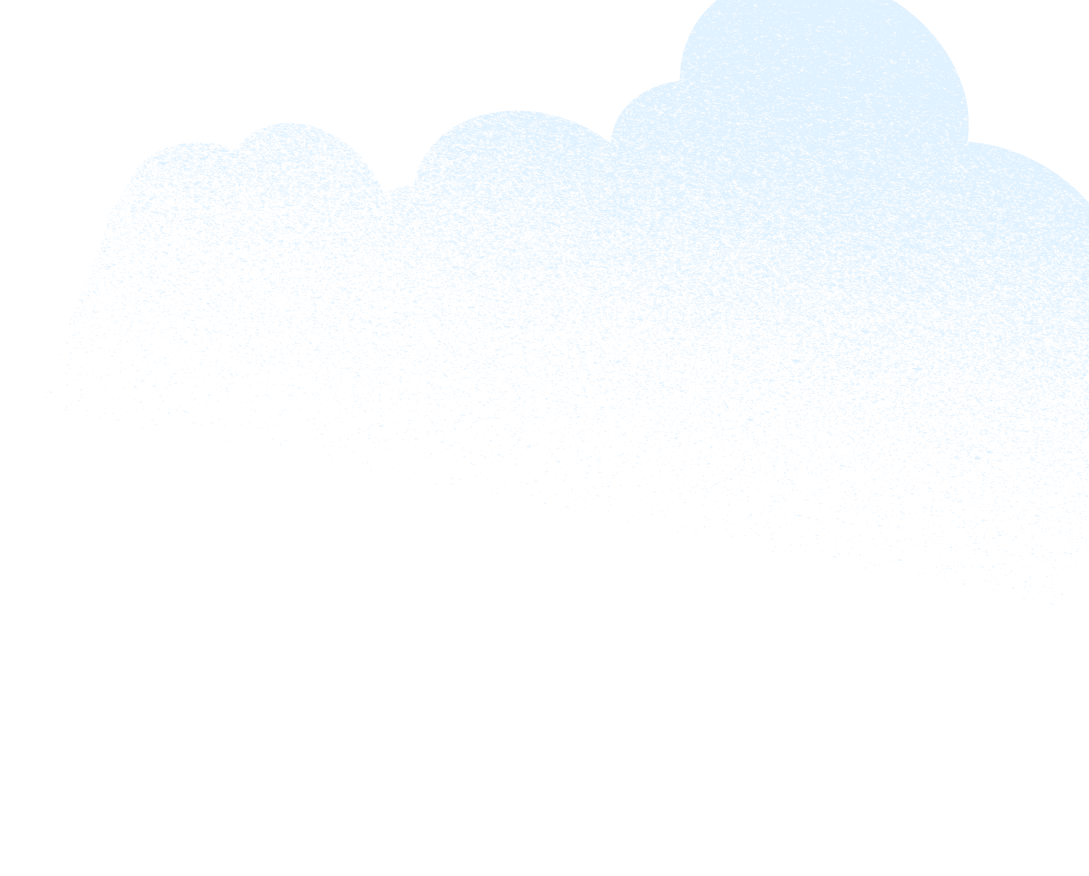