How to Boost Government Efficiency with AI and Personalisation?

Transform public sector operations with AI-driven personalisation for enhanced efficiency and satisfaction. Read on to learn more!

Challenges running central government services today
Central Government runs complex services that must scale to support the needs of a country’s population. Services like tax collection or benefits are operated in perpetuity, adhering to policy and legislation established over many years.
Governments around the world have moved towards a digital self-serve-first approach in recent years. The success of digital self-service reduces the level of staff assistance required in, for example, a contact centre. High levels of staff-resourced assistance make services more expensive to operate.
Departments seek solutions that maximise citizen self-service through:
- Digital government services via online channels
- User journeys that maximise the likelihood of a user achieving what they set out to do
- Transposition of complex legislative policy to easy-to-understand guidance for citizens
Solutions that guide the user through a digital process successfully make government services better for users and cheaper for the taxpayer. Is Artificial Intelligence (AI) a good candidate to enhance the digital services that governments provide?
The AI opportunity for government services
There are two broad types of AI to consider:
- Predictive AI utilises machine learning to analyse historical data and identify patterns to forecast future events. It has the potential to improve decision-making by predicting trends and behaviours, and has value for strategic planning. For example, government services can better predict outcomes by factoring in diverse datasets. This might include weather and staff sickness data to augment historical peak-period data, predicting the likelihood of staff shortages.
- Generative AI creates new content, ranging from text and images to computer code, based on its training data. It learns from vast datasets to generate original outputs that mimic human-like creativity. It has the potential to produce contextually relevant material and enhance understanding of complex information. Given the complex nature of policy in many government services, training Generative AI on policy data enables the creation of summarised output specific to a user’s needs. Instead of designing a single set of guidance that hopes to cover the broad needs of a population, generative AI could summarise guidance at runtime of a process tailored to the individual user’s needs.
In either type of AI, it’s a commonly accepted principle that the larger and more comprehensive the datasets are, the more accurate the output will be. As government services run for decades, there are characteristics of government data useful to an AI training dataset:
- Volume – data is collected on a national scale across sectors such as taxation, benefits, healthcare, and education. AI algorithms, particularly those based on machine learning, thrive on large datasets to make accurate predictions.
- Variety – similar data points are captured across diverse populations, for example, taxation statistics from dense metropolitan-based cohorts to isolated rural communities. For AI to provide accurate and relevant outputs, it must understand multifaceted issues holistically using datasets that are as representative as possible.
- History – Departments have gathered data about citizens’ behavioural patterns for decades. AI models must be trained to recognise long-term trends and changes, particularly where they may be deployed to influence policy or forecasting.
Artificial Intelligence in the Public Sector
Why is AI a good candidate to enhance the digital services that the government provides?


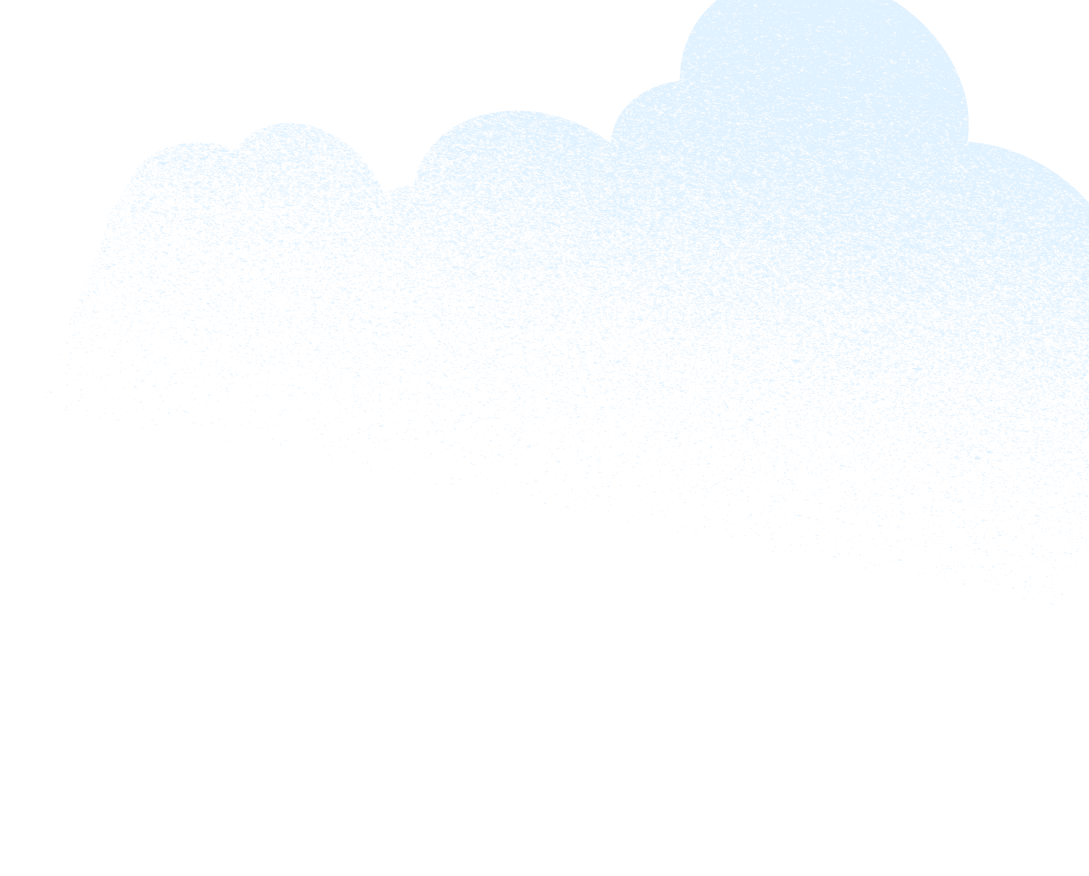
The risks of the AI opportunity
Like many technology innovations of the past, AI will only be successful if it is trained on accurate and useful data, and is applied proportionately to the task at hand. Consider the following characteristics of data found in many government departments:
- Poor quality – data collected over long periods of time is more likely to be stale or incomplete. AI trained on incomplete data will create misleading outputs and incorrect conclusions.
- Lack of standardisation – data the government collects often sits in siloed, single-purpose systems with multiple formats and schemas. A lack of interoperability and integration makes it challenging to feed AI models with harmonised datasets.
- Regulations – Government data is often governed by regulations that limit its use to the specific purposes for which it was collected. Such restrictions influence a department’s willingness to share datasets, leading to AI models that analyse behavioural trends about a citizen based on limited inputs.
Applying AI safely and transparently is critical to building public trust in the outputs AI provides. Like any technology implemented for the first time, this involves rolling out controlled beta versions of the product with transparent outcomes shown to users. It also means applying ‘human in the loop’ patterns, where AI output is assessed by a human before being applied.
Applying AI to a Government use case
Consider a welfare service provided by a central government department. Welfare must meet the diverse needs of an entire adult population while including appropriate checks and balances to dismiss fraudulent claims.
AI could augment the welfare process through:
- Better outreach: localised economic or social data is used to create highly targeted segments of a population using predictive AI. Generative AI, trained on government policy and previous outreach efforts, communicates with these targeted segments using a style and language specifically tailored to their needs.
- Better self-service: understanding eligibility is complex, and generates high volumes of calls to an inbound contact centre. A digital bot, deployed on the web within a digital service or through a claimant’s channel of choice like WhatsApp or SMS, enables personalised self-service. The bot is trained on historic eligibility cases and policy, and understands and produces natural language. Responses are tailored using a predefined tone of voice, or one similar to the input it receives.
- Intelligent fraud identification: predictive AI is applied to historical welfare applications within the department to identify and flag suspicious data points. Through feedback and tuning of the model, correlation can be identified and prediction accuracy increased, allowing fraud caseworkers to focus only on valid applications.
In these examples, AI augments existing services with human-in-the-loop feedback encouraged at each stage. The department can implement AI benefits in a transparent and controlled way.
GenAI: The Trust Multiplier
Get insights from thousands of people across the globe about the evolution of digital government experiences and the role of AI in government service.


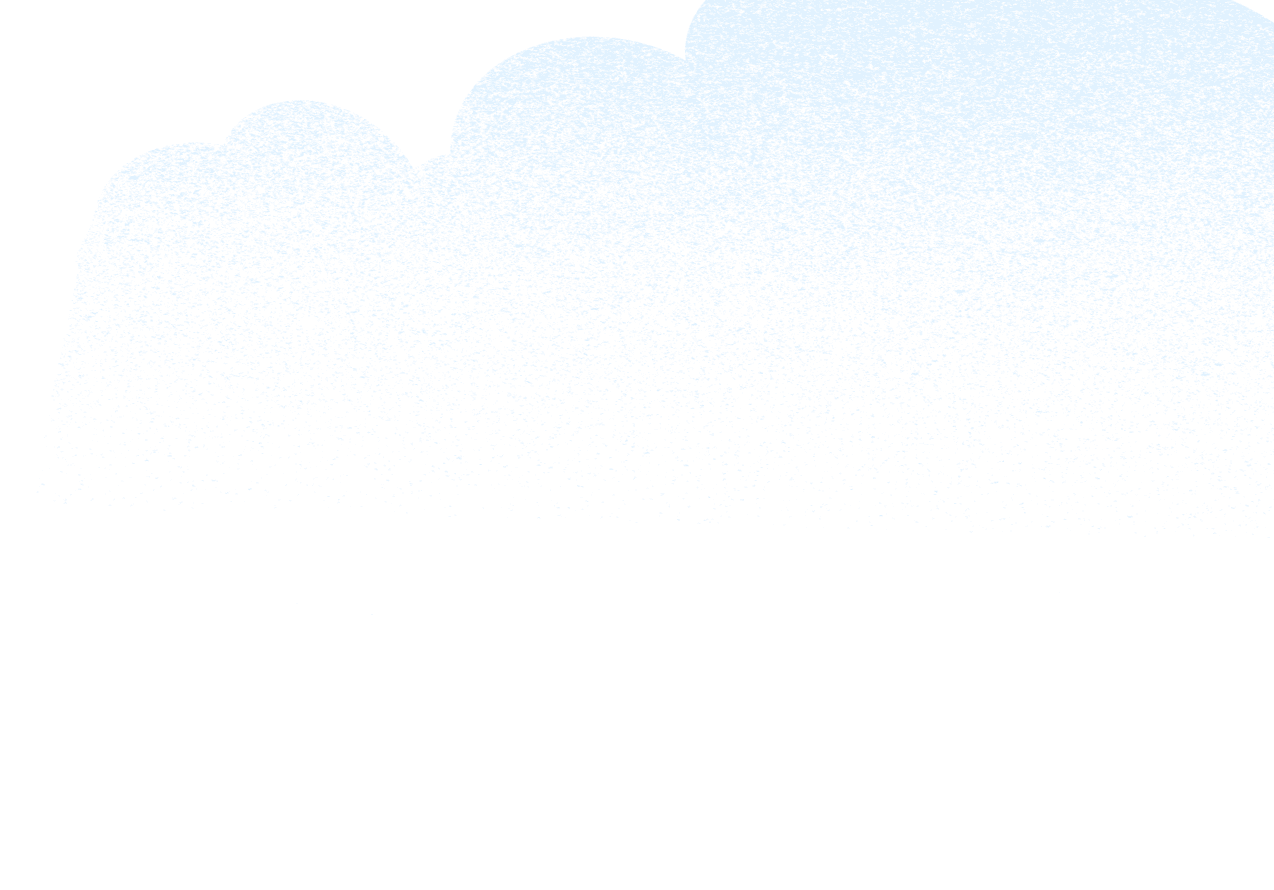
How Salesforce supports a department’s AI journey
Salesforce supports departments to deliver AI benefits transparently through:
- Data integration: with hundreds of out-of-the-box connectors, Mulesoft connects and integrates data sources from live operational systems to data warehouses to ensure all of a department’s data can be ingested by AI models
- Data harmonisation: Salesforce Data Cloud harmonises data sources at the scale and volume governments operate, with standardisation of schemas from siloed systems so data fed into AI models is consistent and trusted
- Data activation: AI outputs, such as targeted cohorts or predictive insights, activate data at the population scale. Outreach is executed on these cohorts through native journeys in the Salesforce Marketing Cloud
- Implementing AI on data: the Salesforce Einstein AI layer has been delivering AI in Salesforce applications for nine years. Creating a predictive model or generative AI prompts is delivered with rapid low-code tools so departments learn fast. AI features are deployed incrementally to a controlled set of users, for example in a private beta phase.
- Keeping a human in the loop: where an AI model produces output within the flow of work, Salesforce keeps ultimate control of the next step with the user. The user is requested to provide feedback on how useful the output was for improved model accuracy.
- Transparency and controls: Salesforce’s Einstein Trust layer ensures confidential data is masked before being sent to external AI models. It maintains a full audit trail of AI inputs and outputs to support fine-tuning and maximising the accuracy of outputs. Data will not be retained by external AI models for retraining.
Salesforce Einstein1 platform supports departments to iteratively deliver AI benefits and build trust, delivering better outcomes for users at a lower cost to the taxpayer.