Did you know that data science plays a critical role in nurturing generative AI? In many ways, generative AI is like an apple tree: you must give it the right nutrients to produce good results. The apple tree needs fertile soil, sunlight, and water to produce delicious fruit — generative AI needs a well-organised data stream to produce quality content. Without these things, your harvest will be poor.
Analytics and IT Leaders know this. The Salesforce State of Data and Analytics 2023 report shows that improving data quality is their number one priority. Now let’s take a closer look at this field and understand how it contributes to building trusted AI. We’ll also explore how data scientists use generative AI in their daily jobs.
- What are AI and data science?
- How does data science help build trusted AI?
- Examples of generative AI for data scientists
What are AI and data science?
You might be wondering why you need to know about AI data science. Yes, it’s technical but important. Actually, a lack of comprehension is hurting the commercial application of data. Our research shows 41% of line-of-business leaders say their data strategy has only partial or no alignment with business objectives. So it’s worth getting to grips with.
First, it’s important to understand the distinction between ‘AI’ and ‘data science.’ AI is a technology where computers ‘think like humans’, performing complex tasks like reasoning, planning, learning, and understanding language. Data science, on the other hand, is a wide-ranging field that uses automated and scientific methods to analyse large amounts of data and derive valuable insights from it.
Now here’s where things get more complicated. As well as being distinct, they need each other and are mutually beneficial. Data science techniques are used to build generative AI, while AI is used to enhance data science techniques. Understanding and using AI and data science can help you build resilience in your organisation. On the other hand, the stakes for not using data are high. In The State of Commerce Report 2023, leaders who report that they aren’t effective at using their data are 37% more likely to report not being prepared to handle rising inflation.
Read our State of Data and Analytics Report
We surveyed over 10,000 analytics, IT, and business leaders for insights on data management and decision-making in the age of artificial intelligence. Discover key insights.


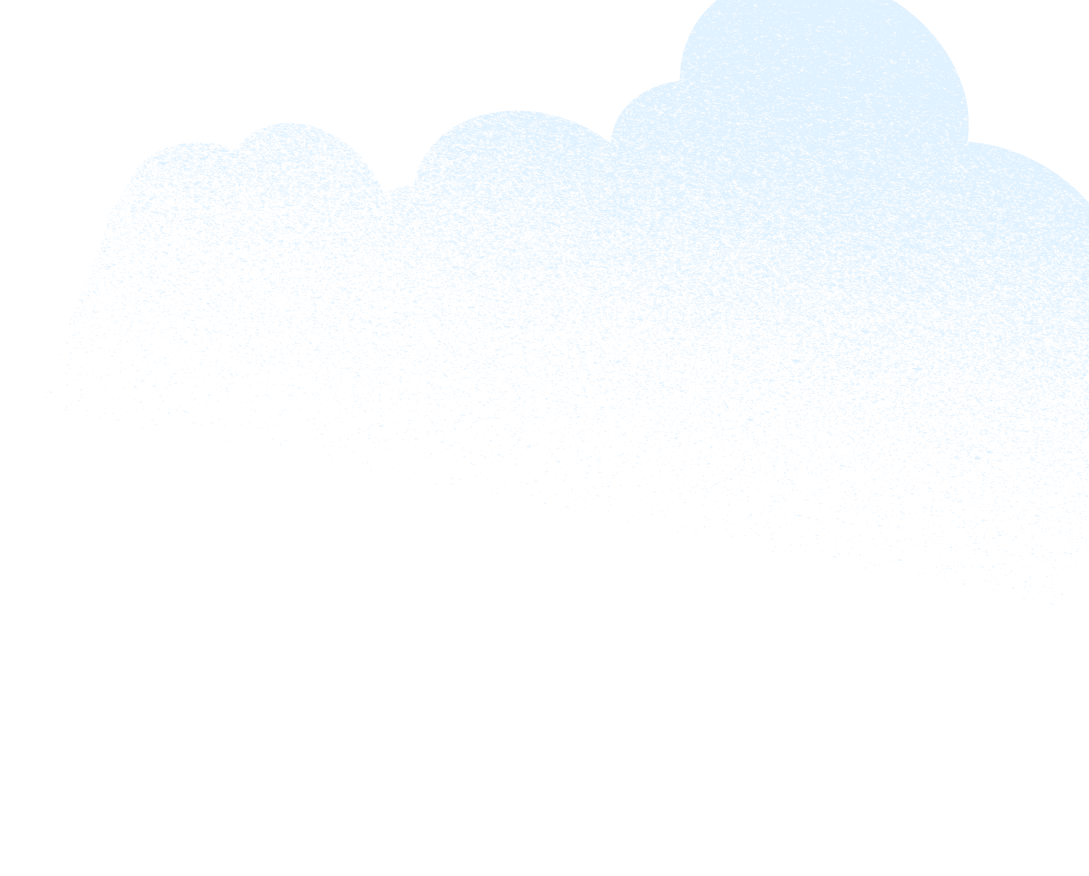
How does data science help build trusted AI?
Generative AI is sometimes called a large language model (LLM). Essentially, it’s a type of AI that’s been trained on a lot — and we mean a lot — of text.
As it’s built on such a large amount of text, data science is required to help get the most out of the data. Your data needs to be structured and organised in a way that allows the LLM to recognise meaningful patterns. Thus, improving its ability to give you coherent and relevant outputs.
If you neglect data science when building AI models, it’s likely the technology will be of poor quality — in other words, the age-old ‘Garbage In, Garbage Out’ principle will come into play. This states that if the input data is of poor quality, the output might be biased, discriminatory, misleading, irrelevant, or useless.
And this is a big concern. In fact, in our The State of Data and Analytics report 2023, 87% of analytics and IT leaders said that advances in AI make data management a high priority, while 92% said that the need for trustworthy data is higher than ever.
To ensure generative AI success, it’s imperative you have complete, up-to-date, accurate, and quality data. At Salesforce, we’ve a large team of data scientists working on Einstein, the first generative AI for CRM, who help us do just that. Through their efforts, we’ve built the following safety features into our platform:
- Dynamic grounding: This steers an LLM’s answers using the correct and most up-to-date information, “grounding” the model in factual data and relevant context. This prevents AI hallucinations, or incorrect responses not grounded in fact or reality.
- Data masking: This replaces sensitive data with anonymised data to protect private information and comply with privacy requirements. Data masking is particularly useful in ensuring you’ve eliminated all personally identifiable information like names, phone numbers, and addresses when writing AI prompts.
- Zero retention: This means that no customer data is stored outside Salesforce. Generative AI prompts and outputs are never stored in the LLM and aren’t learned by the LLM. They simply disappear.
These are a couple of examples of how data science can build trusted AI. Now we’re going to show you things the other way around, focusing on how generative AI can help data scientists in their daily jobs.
Examples of generative AI for data scientists
By using generative AI, you can build models that automatically learn patterns and relationships within data. This allows predictions and the discovery of insights. Here are two of the most powerful use cases.
- Data storytelling: Finding value in data, telling a story with it, and presenting findings to senior leadership is a key part of a data scientist’s job. Generative AI can make this simpler by identifying the insights that data scientists need more quickly, helping them generate better visualisations in less time.
- Predictive analytics: Where generative AI produces new outputs based on recognised patterns, predictive analytics uses historical data to make informed decisions about the future. By integrating generative AI into the latter process, data scientists can make their models more accurate. For example, generative models can be used to create synthetic data for scenario testing, enabling predictive analytics to factor in additional variables and datasets, leading to more insightful and accurate forecasts.
Reap what you sow: Why generative AI success starts with well-organised data
Ask any data scientist what they spend most of their time on, and you can bet they’ll say something along the lines of “getting data in shape.” Rightly so. Without a foundation of good, well-organised data, it’s impossible to build successful generative AI. And it’s important to remember the crucial role that data science plays in making this possible.
You’re probably well aware that you’ll be using AI in some form over the next few years. In fact, AI will be (and in many cases, already is) built into many of the platforms you currently use — for example, Salesforce — so you’ll be engaging with AI even without extra investment. Whether you use AI tools built with the help of data scientists, or build a data science team, hopefully this blog has given you a valuable foundation of knowledge.
Discover the generative AI for CRM.
Want your customer data to create customisable, predictive, and generative AI experiences to fit all your business needs safely? Bring conversational AI to any workflow, user, department, and industry with Einstein.
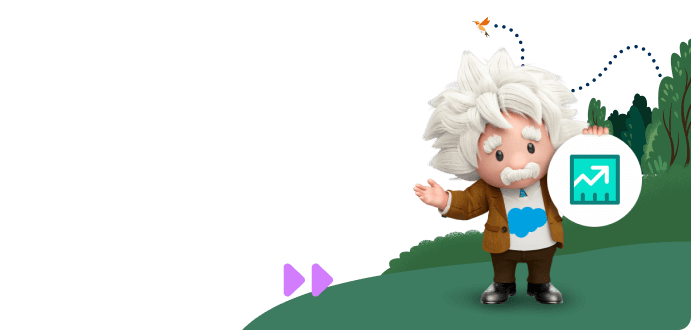
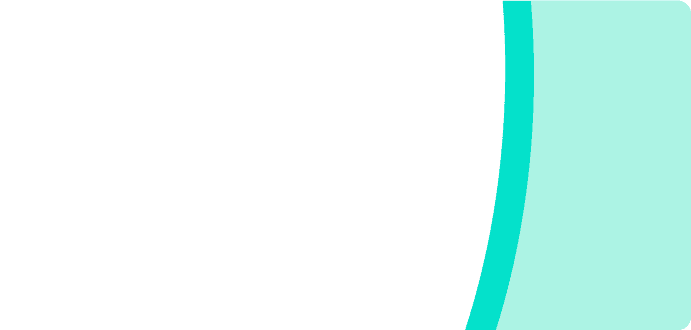