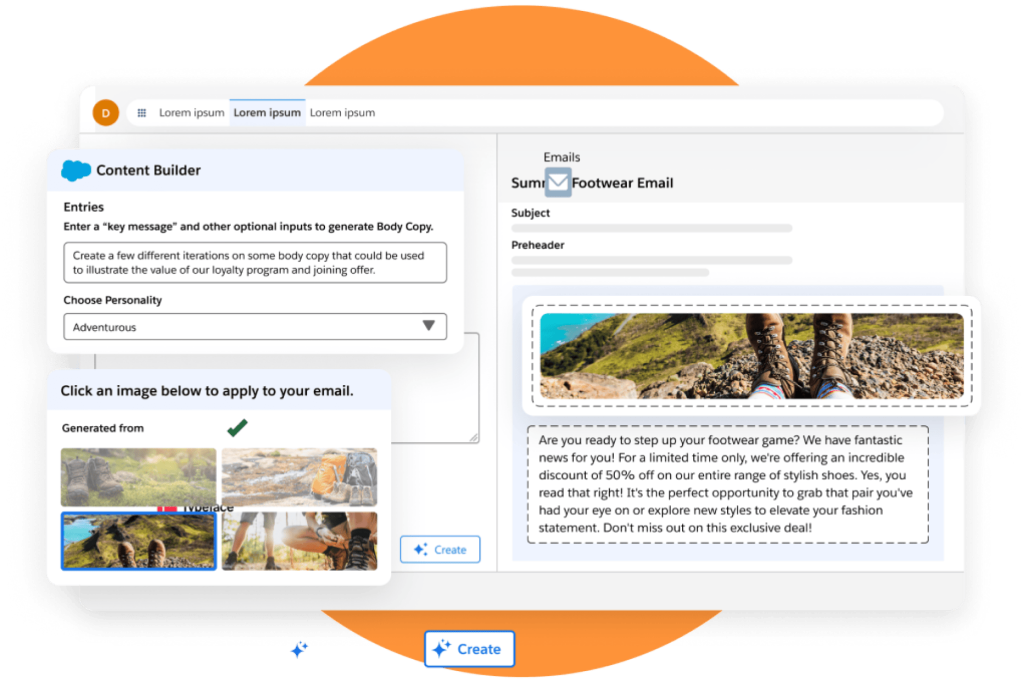
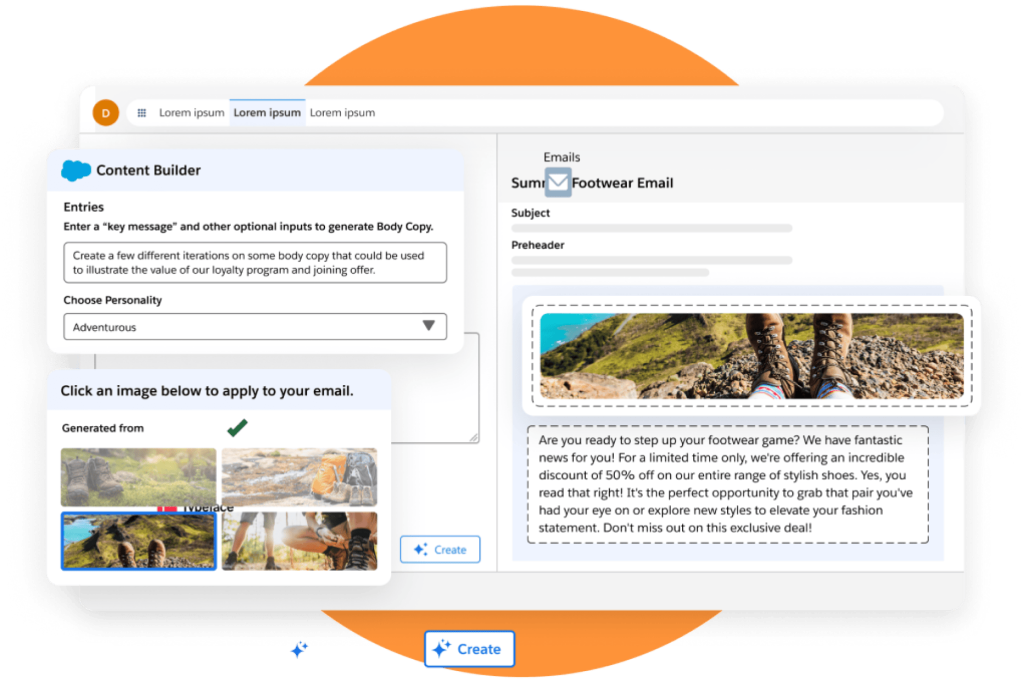


Blue or yellow? “Learn more” or “Read more”? Send that email on a Monday or on a Wednesday? While trial and error are one way to determine the best possible outcome from your digital marketing efforts, A/B testing, also called split testing, is faster, more efficient, and able to produce hard data to help your team make informed, effective decisions. Read on to learn how to implement A/B testing to your marketing efforts.
Definition of A/B testing (also called Split Testing):
A scientific approach of experimentation when one or more content factors in digital communication (web, email, social, etc.) is changed deliberately in order to observe the effects and outcomes for a predetermined period of time. Results are then analyzed, reviewed, and interpreted to make a final decision with the highest yielding results.
Production releases don’t happen at LinkedIn without split testing. Netflix
is notorious for running experiments on their sign-up process and content effectiveness, and they encourage their designers to think like scientists
.
A/B tests can improve operational efficiency. Supported by data, the right decision can become apparent after about a week of recorded outcomes. Testing, rather than guessing, yields valuable time for creative teams, marketing teams, and operational associates to work on other priorities.
The financial and opportunity cost of making the wrong decision can be minimized with A/B testing, not to mention that the learning gained during the test can be invaluable. If you’re testing a blue button against a yellow button split evenly among your audience, and testing reveals that the button should indeed be blue, the risk of exposing the less effective experience is cut by half.
Some of the most innovative companies in the world rely on A/B tests for marketing and product decisions. Experimentation is so integral to some businesses that they developed their own customized tools for their testing needs. Production releases don’t happen at LinkedIn without split testing. Netflix
is notorious for running experiments on their sign-up process and content effectiveness, and they encourage their designers to think like scientists
. Google
conducted 17,523 live traffic experiments, resulting in 3,620 launches in 2019. Jeff Bezos famously said
, “Our success is a function of how many experiments we do per year, per month, per week, per day.”
Why use split testing?
When running an A/B test on a webpage, traffic is usually split between some users who will see the control, or the original experience (for example, the blue button), and those who will see the variation, or the test experience (the yellow button). Unlike qualitative testing or research where users tell us what they will do, during an A/B test, data are collected on what the users actually do when choosing between the control and the variation.
Without going too deeply into the mathematics about how to conduct an A/B test, there are two foundational principles that everyone should understand about experimentation: random selection and statistical significance.

See the top trends in data, AI, and more — from nearly 5,000 marketers worldwide.
What is random selection in A/B testing?
In order to have confidence in the results, users who are shown the variation should be representative of the targeted user base – for example, all users should be people in the market to buy a pair of boots. In most cases, the number of users are split evenly between control and variation. This is what we mean by random selection, and this is usually employed in testing to avoid any bias. Note, however, that sometimes only a small portion of users are selected to see the test variation to minimize risk.
''Our success is a function of how many experiments we do per year, per month, per week, per day.''
Jeff Bezos, CEO of Amazon
Most commercial software capable of running A/B testing in different marketing channels (including Marketing Cloud) normally has random selection functionality built-in so that marketers and non-technical people can execute tests easily.
What is statistical significance in split testing?
Statistical significance is a measure of the probability of an outcome – whether it is accurate or simply due to luck or random chance. For example, if an analyst says that the test result of 5% increase in conversion rate has a statistical significance of 90% confidence, it means that you can be 90% sure that the test results can be trusted.
Analysis and research inform effective testing
Albert Einstein once said, “The formulation of a problem is often more essential than its solution, which may be merely a matter of mathematical or experimental skill.” The first and most important step in an experiment is to identify key problems (or measurable business goals) and validate them through analysis and research.
Let’s say a web team learns that they have an underperforming landing page. Rather than jumping right into solutions and random experimentation changing images, messaging, or layout, the team needs to first look at data to identify the problem. Looking at page analytics and user data, they identify that the issue is from the primary call to action (CTA). This type of thoughtful, purpose-driven research is the analysis necessary to set up the A/B testing process.
Generate ideas and create a hypotheses
Once the problem is identified and validated, start generating solutions that will solve the business problem. This should be accompanied by hypotheses on possible results and impact.
As a side note, it’s important to distinguish the difference between ideas and hypotheses. An idea is an opinion on the what, where, who, and how of an experiment. Hypothesis is the reason why the idea, if implemented, will yield better results toward the goal than the current state. For example, to change the color of the button to yellow is an idea. The belief that the high contrast between the color of the button and background will help users notice the button and result in higher clickthroughs is then the hypothesis.
Build lasting relationships that drive growth with Marketing Cloud.
Action all your data faster with unified profiles and analytics. Deploy smarter campaigns across the entire lifecycle with trusted AI. Personalize content and offers across every customer touchpoint.
Prioritize and sequence to determine what to test, when
When trying to solve a digital communications problem, it’s likely that there are many tests you’d like to run, and that there are several hypotheses you’d like to put to the test. However, to get clean testing data, you can only solve for one hypothesis at a time.
This is when you should start prioritizing and sequencing the tests. Most successful experimentation programs weight these decisions based on strategic importance, effort, duration, and impact. Some larger programs assign a score to each of the criteria. The proposed test that has the highest score gets to the front of the line. That said, no scoring system is perfect, and they should all be refined over time.
Finalize your testing plan
Once you know which test you want to run, it’s best to develop a robust test plan prior to building out the test. A comprehensive A/B test plan should include the following information:
- The problem you’re trying to solve
- A SMART (Specific, Measurable, Achievable, Relevant, and Time-based) goal
- The hypothesis being tested
- Primary success metrics (how the results will be measured)
- Audience (who you’re testing, and how many)
- Location (where the test will be conducted)
- Lever (what changes between the control and the variation)
- Duration of the test to achieve its predetermined statistical significance – duration will be impacted by whether the split is 50/50 or 80/20 (which is determined by your risk tolerance)
A well-documented plan provides transparency which helps you gain alignment across the organization, and it helps reduce collision with any other test. This is especially important if you work in a larger organization where many teams can be conducting tests simultaneously.
Build out your A/B test
With an approved test plan, it’s time to start building the test. If you are changing the button color, image, or text on a web page, execute using specialized testing software and services. Products such as Marketing Cloud Personalization in Marketing Cloud allows users to change colors for an A/B test using a visual editor so that no coding skill is required. You can also test email subject lines right in the product.
If there are any customer-facing elements, it is a good idea to work with design/UX to make sure that changes comply with brand and accessibility standards at your company. If it is a more complex test where code changes are involved, have your software development team do a code review before launching the test. And if your company has the resources, run everything through quality assurance before moving forward.
Execute and monitor your split testing
Once you hit the launch button, the job is only halfway done. Experiments need to be monitored regularly to ensure they run properly, especially if you’re running a test for a significant amount of time. Sometimes a change in the position of a component being tested will cause the testing tool to stop allocating traffic to the variation. Backend production releases might cause the testing tool to function improperly or stop sending data to and from the testing tool. Regular monitoring will ensure breakage is caught as soon as possible. A data visualization tool such as Tableau can be helpful to combine data and provide a more holistic view of the test.
In traditional A/B testing, you set a test duration, and then you don’t stop the test until it reaches that day and/or the volume you’ve set. However, if the interim result is highly skewed in one direction (i.e. the test variation’s conversion rate is consistently much lower than the control and there is a strong indication that it will not improve over time), it becomes a business decision whether end the test early in order to preserve the health of the business.
Learn from analyzing the A/B test results
An experimentation report should highlight the meaning behind the data. Analysts for the experiment should interpret the data objectively and “tell the story” behind the numbers qualitatively. More importantly, recommendations on steps to gain a deeper understanding of user behavior should always be provided. Those recommendations can be the basis of the next experiment.
And with that, the cycle of iterative testing and learning goes full circle with the next test underway.
What’s next?
Keep in mind that a split test is not a failure if a test did not turn out as you’d thought. It’s merely that the hypothesis has not been proven statistically. It is unreasonable to believe that your ideas and practices will be right 100% of the time. With the right test design, execution, monitoring, and analysis, you will always learn something in the process. That’s always a win.

Stay up to date on all things marketing.
Sign up for our monthly marketing newsletter to get the latest research, industry insights, and product news delivered straight to your inbox.