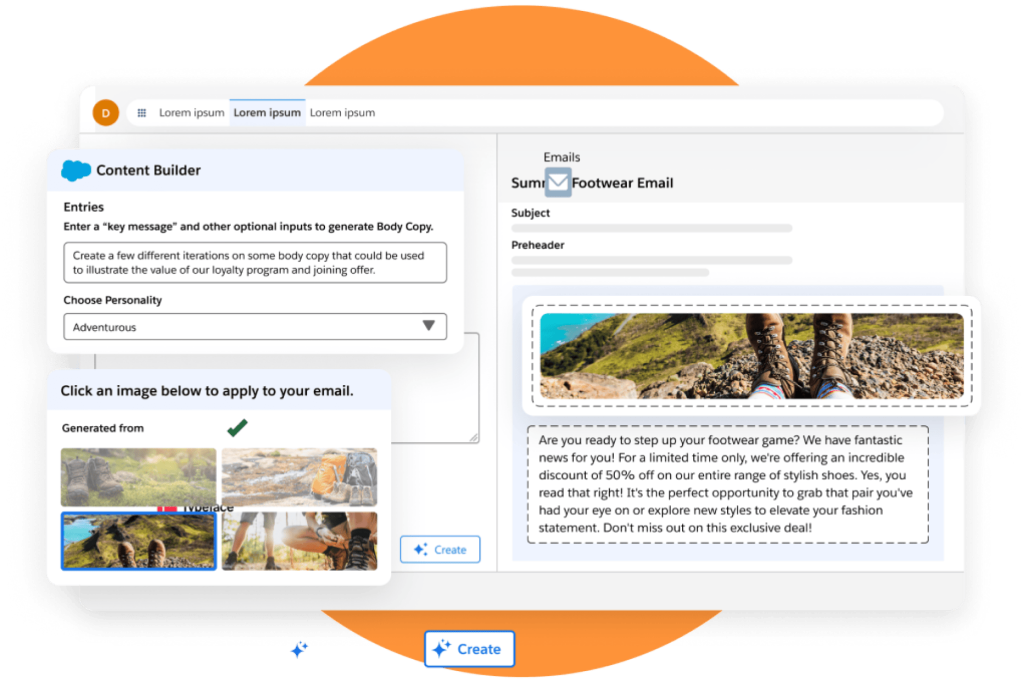
A complete guide to email marketing A/B testing
What is email A/B testing and what’s the best way for your marketing team to do it? Here’s a handy guide to get you started.
What is email A/B testing and what’s the best way for your marketing team to do it? Here’s a handy guide to get you started.
Comparison isn’t always the thief of joy, especially when it comes to email marketing. When’s the right time to hit ‘send’? What subject line should you use? Which image is best? These might seem like small choices, but they are things that email A/B testing can help you to figure out.
According to our State of the Connected Customer report, nearly two-thirds of customers expect companies to adapt to their changing needs and preferences. Gauging results of an audience with a single email can be difficult. But sending multiple versions of an email - with different subject lines, preview text, copy, calls-to-action or timing of delivery - can give you a basis for comparison. With A/B testing and the right email marketing tools in place, you get all the valuable data you need to improve your next round of outreach
In this blog, we’ll guide you through how email A/B testing works, including its challenges, best practices and ways artificial intelligence (AI) can help.
Email A/B testing is a marketing strategy where you provide different versions of a campaign to your audience. The “A” version is displayed to some of your audience, while another subset gets the “B” version. It can be anything from subject lines to body copy to offers to images.
Email A/B testing can help you to optimise your campaigns. Testing two variations of an email will provide insights to see which version your audience likes the best.
There are a lot of reasons why email is one of the easier methods for A/B testing. The main one is that email A/B testing includes binary responses - clear-cut, two-option reactions or actions recipients can take. These include things like clicking or not clicking a link or opening or not opening an email. Email is a good candidate for A/B testing for its ability to test the following:
Let’s break down the way email A/B testing works a little further.
Selection of variables: Marketers choose specific elements of the email to test. Common variables include the subject line, sender’s name, email content, CTA buttons, images, headlines and more.
Creation of variations: Different versions of the email are created - each with a single variable changed. For example, if testing the subject line, you might create two versions of the email with different subject lines but keep the rest of the content identical.
Random assignment: The email list is divided randomly into groups, with each group receiving one of the email variations. This ensures that the test is conducted with a representative sample of the audience.
Distribution: The email variations are sent to their respective groups within the same time frame to minimise external factors affecting the results.
Data collection: As the emails are opened, clicked and acted upon, data is collected on the performance of each variation. Marketers track metrics like open rates, click-through rates, conversion rates and revenue generated.
Analysis: After a predetermined time, the results are analysed to determine which variation was more effective in achieving the desired goal. The winning variation is then chosen.
Optimisation: Based on the results, marketers can apply the winning elements to future email campaigns and continue to refine their email marketing strategy.
Sending two (or three or ten) versions of the email will provide you with lots of data to work with. This kind of data-driven approach is a way to increase open rates, click-through rates, conversion rates and other key performance indicators.
While trial and error is one way to determine the best possible outcome from your email A/B testing, these methods will help your team make more informed decisions.
Remember, taking the long view and being patient during the process is key.
Building tests based on the best available data will ensure that performance metrics, customer preferences and industry benchmarks are taken into account. And, learning from your results involves a ton of data analysis. Make sure to take the time to delve into the nuanced details of each A/B test, looking at the overall outcome and any subgroups that displayed notable behaviour.
View individual results as a piece of a puzzle - and the large view when you’re “done” putting the pieces together is a deeper understanding of your audience’s preferences and behaviours.
Integrating insights into your future A/B testing strategies will help you refine your strategies so you can maximise the effectiveness of your email campaigns.
Email A/B testing has its own set of issues. One major hurdle to clear is making sure your testing aligns with your campaign objectives. As marketers, we know that any strategy demands consideration of metrics that reflect success. Now is the time to ask yourself how you want to implement and analyse your strategy. Did a subject line fail because it ended in a question mark and the other one didn’t? Or was it simply because the verbiage was bad?
Ensuring an adequate sample size is another challenge. Without it, your results may not be representative of the broader audience. You risk misinterpreting results when data is inaccurate.
Remember, the success of your email A/B testing doesn’t hinge on a single factor; rather, it depends on the interplay of all elements - such as content, design, audience preferences. Taking a holistic view is critical for drawing adequate conclusions.
The beauty of A/B testing is its snowball effect. As you refine messaging, you gradually sculpt communications into their most successful versions. This process helps uncover nuances that resonate with your audience. Those improved messages lead to better data, which lead to even more improved messaging, which leads to even better data.
The result is better open rates, increased click-throughs and ultimately, elevated conversion rates. Through continued testing, each email that reaches your subscribers is crafted to achieve a bolstered performance.
Action all your data faster with unified profiles and analytics. Deploy smarter campaigns across the entire lifecycle with trusted AI. Personalise content and offers across every customer touchpoint.
You can take these six steps to get your email A/B testing programme off the ground.
It’s important to ask yourself if you can support the demands of A/B testing. Without a solid infrastructure, there’s no guarantee you can track and analyse results. Take the time to set yourself up with a testing process that makes the most of your results.
AI is all the buzz these days - and for good reason.
Predictive AI, for example, can serve as a force multiplier for A/B testing things like send times. AI can determine the optimal time to send emails to each recipient based on their past behaviour. This increases the chances of emails being opened and read. It helps you refine your macro strategy as you learn about specific customer tendencies. This allows you to get more accurate with future segmenting.
Generative AI, on the other hand, makes it faster to create scalable variant content for testing, allowing you to test bigger changes without having to generate two entirely different sets of copy from scratch. Generative AI models can assist in generating email content, including subject lines, body text and personalised recommendations. This can help you to create compelling and personalised emails for A/B testing, saving time and resources in content creation.
It’s worth it to familiarise yourself with these definitions as you consider all the new ways AI can inform better results:
Generative AI for content creation: Generative AI models can assist in generating email content, including subject lines, body text and personalised recommendations. This can help you to create compelling and personalised emails for A/B testing, saving time and resources in content creation.
Segmentation and personalisation: AI can analyse vast amounts of data to segment email lists based on various factors like demographics, behaviour and preferences. This allows for highly targeted and personalised email content, leading to better A/B testing results.
Predictive analytics: AI can predict which email variations are likely to perform better for specific segments of your audience. It uses historical data to make these predictions, making email A/B testing more efficient.
Content optimisation: AI tools can analyse email content and suggest improvements based on historical data and best practices. This can help you to create more engaging and effective email content for A/B testing.
Send time optimisation: AI can determine the optimal time to send emails to each recipient based on their past behaviour. This increases the chances of emails being opened and read.
Subject line optimisation: AI can help to generate and test subject lines to find the ones most likely to capture the recipient’s attention and increase open rates.
Dynamic content: AI allows for dynamic content within emails, where the content changes based on the recipient’s behaviour or preferences. This can improve engagement and conversions.
Predictive scoring: AI can assign predictive scores to email recipients to indicate their likelihood of opening, clicking or converting. You can use this data to prioritise your email A/B testing efforts.
Automation: AI can automate the A/B testing process by continuously testing and optimising email campaigns in real-time, without the need for manual intervention.
Multivariate testing: AI can handle more complex multivariate testing, where multiple elements within an email are simultaneously tested.
Natural language processing (NLP): AI-powered NLP can analyse the sentiment and tone of email content to ensure that it aligns with your brand’s messaging and is more likely to resonate with your audience.
A/B testing insights: AI can provide insights and recommendations based on A/B testing results. It can identify patterns and correlations that might not be obvious to human marketers.
Feedback analysis: AI can analyse the feedback and responses to email campaigns, helping you to understand the reasons behind success or failure and suggesting improvements.
Email A/B testing is poised for a transformative journey, especially with the expanding role of AI.
Predictive and generative AI will provide marketers with more and more avenues for stronger customer engagement. With predictive AI, you can better anticipate customer preferences, which allows you to tailor A/B tests to individualised segments. Generative AI holds the promise of creating tailored content variations based on intricate customer data. The result is a more nuanced and effective A/B testing process - and tangible business outcomes.
On top of that, advancements in AI analytics will yield more actionable test results. Data-driven email A/B testing has the power to deliver the targeted content that customers demand. Welcome to a new era, where personalisation - and comparison - wins the day.
Sign up for our monthly marketing newsletter to get the latest research, industry insights, and product news delivered straight to your inbox.